Researchers recently produced an algorithm that could guess whether heart patients had lived or died from their condition within a year. By looking at data from a test of the heart’s electrical activity known as an electrocardiogram or ECG, the algorithm successfully predicted patient survival in 85% of cases. But its developers couldn’t explain how the algorithm did this. Its stated purpose was to find previously unknown information that doctors couldn’t see in ECGs.
Developed by US healthcare provider Geisinger, the algorithm was trained using 1.7 million ECG results from 400,000 patients, including some who had died of heart conditions, and others who had survived. But whether the algorithm can be applied as accurately and fairly to predict new cases as it can with this historic data hasn’t yet been tested. The developers have said trials need to happen to see if similar accuracy levels can be achieved with prediction. While this kind of algorithm has lots of potential, there is reason to remain wary of rushing to use these types of artificial intelligence (AI) systems for diagnosis.
One reason to remain cautious about the algorithm’s findings is because it’s very common for algorithms trained using historic data to become biased. This is because much of the historic data currently used to train algorithms can be overwhelmingly from male and white subjects, which can affect its accuracy. For example, algorithms that could predict skin cancers better than dermatologists turned out to be less accurate when diagnosing dark-skinned people because the system was predominantly trained with data from white people.
Historic data can also contain biases that reflect social disadvantages rather than medical differences, such as if a disease is more common among a minority group because they have worse access to healthcare. Such bias is not just found in health-related algorithms, but also algorithms for facial-recognition and photo-labelling, recruitment, and policing and criminal justice.
As such, the Geisinger algorithm needs further testing to see if prediction rates are similarly accurate for a range of people. For example, is it equally accurate at predicting risk of death for females as it is for males? After all, we know that men and women can have different heart attack symptoms, which can be seen in ECG results.
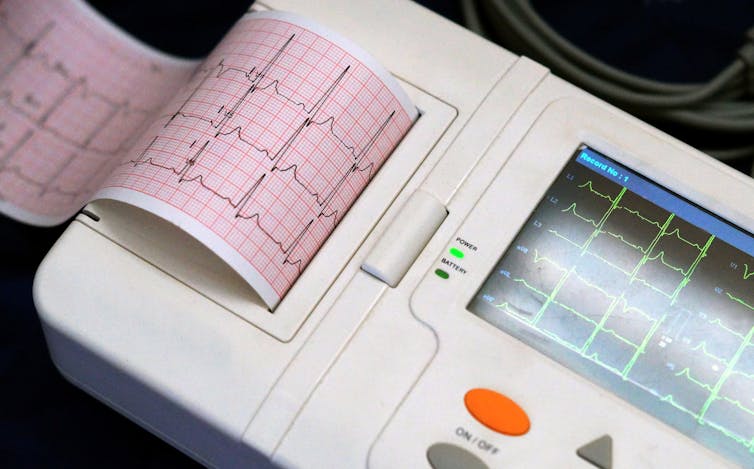
The Geisinger model is also a “black box” system, meaning the decisions it makes can’t be explained by experts and so may have biases that its developers don’t know about. While many researchers and policy makers feel it’s unacceptable to develop “black box” algorithms because they can be discriminatory, the speed with which many algorithms have been developed means there are currently few laws and regulations in place to ensure that only unbiased fair AI models are being developed.
One solution could be to create “explainable AI” (XAI). These are systems designed to allow researchers see what key data features an algorithm is focusing on, and how it reached its decision. This may help them minimise any biases the algorithm may have.
Other guidelines and standards can also help researchers develop fairer and more transparent AI. The IEEE P7003 standard shows developers how to ensure they identify all affected groups in a data-set, test for any bias, and suggest how to rate and mitigate risk of bias. IEEE P7001 guides how to make an AI transparent and explainable.
Understanding the algorithm
Knowing how the Geisinger algorithm makes its decisions is also important so doctors can understand any new features of heart disease risk that the model may have discovered. For example, another algorithm that analysed images to detect hip fractures made its decisions by concentrating on additional clinical data given to it. This revealed the importance of factors such as the patient’s age or whether a mobile scanner was used (indicating the the person was in too much pain to travel to the main scanner).
Research has shown that looking at both the images and the clinical data makes for more accurate diagnoses. But, if researchers can’t explain how the algorithm made its prediction, it might mean the algorithm can’t be developed more for later use in diagnosis.
If doctors are unaware of the features that an algorithm looks at, they might include those features in their own analysis as well as their algorithm’s findings. This would effectively count the features twice, over-emphasising their importance and potentially even producing a misdiagnosis. Doctors could also become over-reliant on the algorithm, might interact less with patients, and could potentially affect doctors’ overall skill levels.
For example, researchers that designed an AI to diagnose childhood diseases (such as bronchitis and tonsillitis) found its diagnoses were better than those of junior doctors. However, senior doctors were still able to make more accurate diagnoses than the AI. So, if not used correctly, such systems could risk doctors never reaching the skill level of current senior doctors.
For this reason, it’s important to consider how such systems are implemented, and whether they’re in line with sector level guidance. Leaving the final diagnosis to a doctor could potentially make an app’s diagnoses more accurate, and prevent deskilling. This would particularly be the case if the model was clearly explainable, and any biases made evident to the doctor.
Although the Geisinger algorithm could predict if someone had survived or not, it’s important to remain cautious of these kind of claims, as AI can contain faults based on how it’s trained and designed. AI systems should augment human decision making and not replace it or health providers. As the Geisinger team advise, this AI has the potential for interpreting ECGs as part of a wider diagnostic toolkit – and is in fact not a way to predict if someone will die or not.
Post a Comment