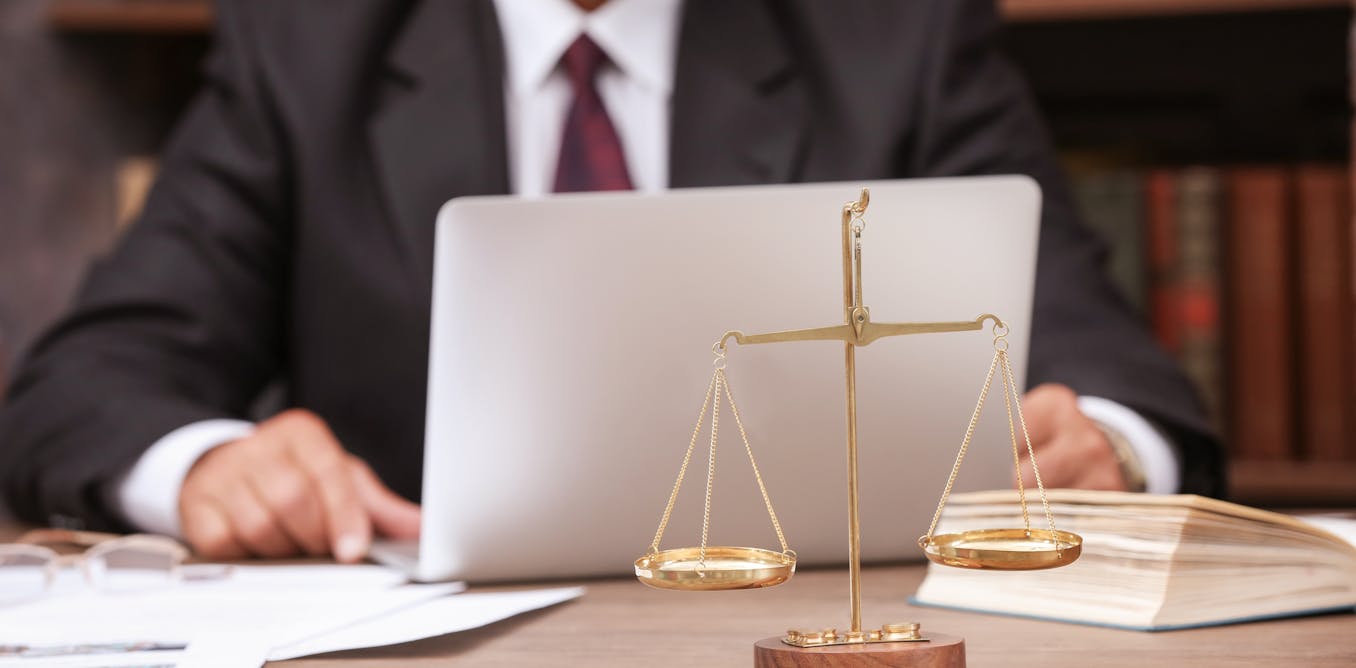
Artificial intelligence is transforming the traditional delivery of legal services.
In general terms, the set of tools broadly called “legal analytics” promises to do two things: increase the efficiency of tasks that once required substantial time and human effort, and mine masses of data to discover new insights that were previously inaccessible.
As legal scholars, we’re excited about the promise of applying these tools to legal research questions. At Georgia State, we’re building interdisciplinary research teams with lawyers and data scientists working side by side. Students are involved too, so that we can educate the next generation of lawyers to leverage these tools in their own practices.
Transforming legal tasks
Suppose that a company wants to forecast which employee complaints lead to lawsuits. Historically, the company might assign a team of analysts and lawyers to comb through complaint records, personnel files and court documents, searching for some pattern that might signal litigation risk. This painstaking process could take months and require an army of people to process thousands of pages of text.
Treating this task instead as a data science problem dramatically improves speed and efficiency. An algorithm could extract key text in bulk and assemble it for analysis. Human time and attention would then be trained only on the relevant information. The labor-intensive search process would be eliminated.
The new generation of analytics tools can do more than simply reduce labor hours. Techniques like machine learning – a type of artificial intelligence where computers can recursively learn from a set of examples without being explicitly programmed to do so – can enable the discovery of new patterns that are beyond the reach of manual analysis. For example, in the scenario above, an algorithm might be able to predict whether any given employee complaint will result in a lawsuit.
At our lab, we are testing the application of analytics tools to a broad range of legal questions. We analyzed all employment lawsuits in the U.S. District Court for northern Georgia to understand which cases win and lose and to identify case features like judges, attorneys and motions that might influence a case’s ultimate outcome.
For example, we found that, when a motion was referred by the presiding district court judge to a magistrate judge for a preliminary report and recommendation, the magistrate judge’s recommendation was the strongest predictor of the judge’s ultimate decision. This raises interesting questions, which we are researching further, about decision-makers’ roles in resolving legal disputes.
Harnessing big data
Legal analytics has captured the imagination of lawyers and researchers alike. In a recent contest in the United Kingdom, 100 lawyers from top London firms were pitted against an artificial intelligence tool to predict the outcome of hundreds of simple financial disputes. The robot won by a wide margin, predicting 86.6 percent of cases correctly, while the humans correctly predicted only 66.3 percent. The tool was “learning” something about the disputes that the humans were missing, beating lawyers at their own prediction game.
Of course, not all legal problems neatly reduce to a set of variables, and human behavior does not always follow detectable patterns. Predictive tools work less well when the relevant dataset is small, or when the text that is subject to analysis is so varied and idiosyncratic that patterns are difficult to detect.
Progress can also bring peril. Historical data about past events often contain bias and inaccuracies, meaning that even the most sophisticated computer code, when fed garbage, can produce only garbage in return. Bail-setting algorithms, for example, have been criticized for perpetuating racial bias in criminal justice.
If lawyers delegate too much of our decisions to algorithms, then we are destined to repeat our historical patterns and mistakes. For instance, litigation prediction algorithms trained on cases from retired judges or outdated case law may miss new developments and recommend an unnecessarily conservative course of action.
In the end, a robot lawyer is a poor substitute for a human lawyer. Human judgment will remain a crucial ingredient in law practice. What will change is when it’s used to augment intelligence gleaned from other systems.
What new lawyers need to know
If the practice of law changes, then that means parts of legal education must change, too.
Some future lawyers will graduate as computer programmers, able to write the code that underlies legal analytics tools. Others will become knowledgeable consumers of the results produced by these tools, able to critically assess the output. Our institution is developing a dual degree in analytics and law, as well as concentrations within the J.D. and LL.M. programs.
We believe that all law schools should wrestle with how to educate today’s students for a future practice. However transformative, in the end, legal analytics is a tool. Tomorrow’s lawyers should be prepared to exploit its advantages, while also understanding where those advantages end and human judgment begins.
Post a Comment